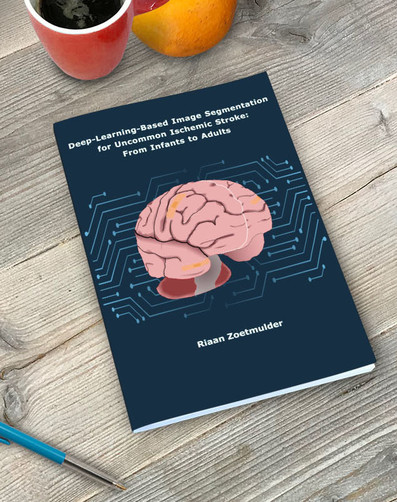
Developing deep learning-based algorithms that accurately segment structures in scans that are relevant to treatment or evaluation of the outcome of uncommon stroke is a difficult task. The difficulty is due to the presence of image artefacts, few data being available to train the networks, and the small volume of some of the target structures. Hence, the aim of this thesis was to investigate, develop, and evaluate deep learning-based algorithms for automatic segmentation of images of uncommon sub-types of stroke. Specific algorithms were developed for posterior circulation stroke lesions and thrombi, and ischemic lesions in perinatal arterial ischemic stroke patients.
Download Thesis