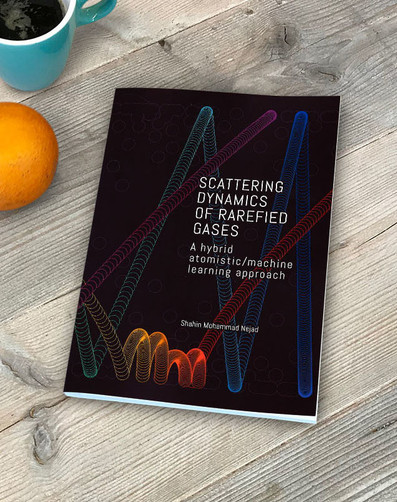
An understanding of rarefied gas dynamics has practical applications in numerous industrial and environmental areas, such as the semiconductor industry, micro- and nano-fluidics, aerospace engineering, nuclear reactor safety, heterogeneous catalysis, and seawater desalination. For these applications, a fundamental understanding of the physics of gas flow is crucial, which, at the design and fabrication stages in these applications, can be achieved using numerical tools that are selected based on the degree of rarefaction in the system and the computational power that can be afforded. In this work machine learning approach is used to create a new model to study rarefied gas dynamics.
Download Thesis