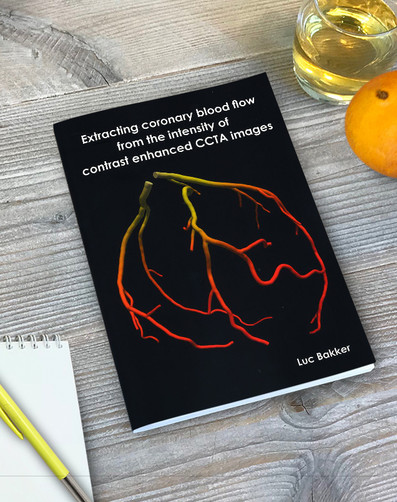
Coronary computed tomography angiography (CCTA) is conducted by injecting an intravenous iodine based contrast material into the bloodstream to highlight the lumen of the coronary arteries. This allows anatomically assessment of the geometrical characteristics of obstructive atherosclerotic plaques, i.e. stenoses. This CCTA can be used to identify the severity of a stenosis and it helps to achieve decisions on clinical intervention. However, CCTA does not provide any information about hemodynamic characteristics such as flow or pressure drops across the stenosis that are better variables to base decisions on.
Over the past years, fractional flow reserve (FFR) has been used in clinical decision making when treating coronary artery disease. FFR is defined as the ratio between the pressure distal to the stenosis and the reference aortic pressure under conditions of maximal flow induced by a vasodilator. Measuring FFR requires fluoroscopic guided invasive catheterization and the insertion of a pressure wire to a location in the vessel distal to the lesion. However, the same metric can be obtained by using computational fluid dynamics (CFD) models. These non-invasive CFD models are created by segmenting a computational mesh of the coronary circulation from the CCTA together with boundary conditions based on scaling laws utilizing patient specific geometry. Although these CFD models have proven to provide a good diagnostic value an improvement could be achieved by inferring the hemodynamic and geometrical information embedded in the contrast intensity found in the CCTA image.
In this thesis a new method for improving these CFD models is proposed called Advection Diffusion Flow Estimation (ADFE) which estimates coronary blood flow from a CCTA image. Additionally a state-of-the-art computational solver is derived and used for validating ADFE. Using this software phantom validation approach, we showed that ADFE is an accurate method to estimate CBF from CCTA images and will hopefully improve FFR derived from CFD models.
Download Thesis