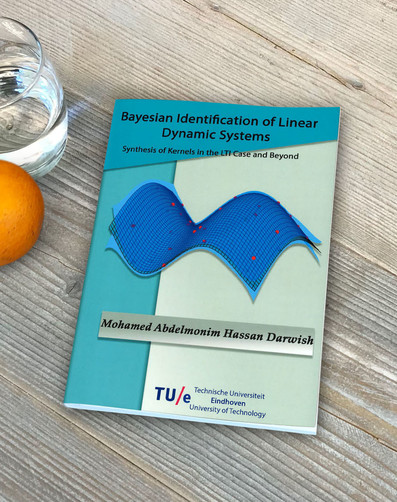
In the last few years, new avenues of data-driven modeling or so-called system identification have appeared due to the introduction of ideas stemming from the field of machine learning. One set of methodologies clustered around the so-called kernel based methods got serious attention in Linear Time Invariant (LTI) system identification due to their interpretation from the Bayesian point of view and their capability to realize an estimator that achieves regularization in Reproducing Kernel Hilbert Spaces (RKHSs). Such achievements are made possible via tailoring these learning techniques to dynamic systems by taking into account dynamic properties as stability. It has been shown that these new regularization based methods may outperform classical parametric approaches, i.e., maximum likelihood and prediction error methods, for the identification of stable LTI systems. The key feature of these learning approaches is that they circumvent the difficulties of model structure and model order selection and introduce a continuous optimization of the bias/variance trade-off based on a nonparametric form of the utilized model structure. The degrees of freedom of the estimation is kept restricted by incorporating prior knowledge of the unknown dynamic system, e.g., smoothness, stability, damping, resonance behavior, etc., through the kernel function that determines the hypothesis space for the estimation problem, i.e., which encodes the utilized nonparametric model structure. Hence, the choice of this kernel function is key to have a successful identification process in terms of a high accuracy model estimate.