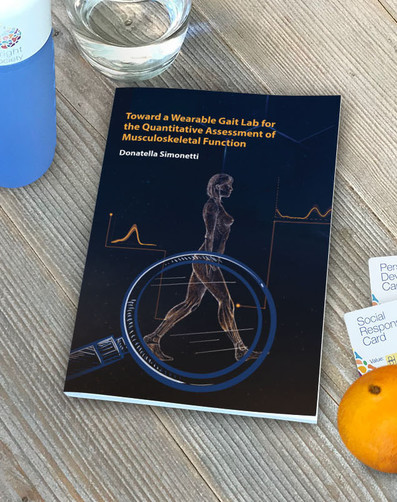
Evaluating musculoskeletal function is vital for understanding movement mechanisms and designing rehabilitation protocols, especially for neurologically impaired individuals like stroke survivors, where restoring locomotion function is paramount for quality of life. In clinical settings, obtaining quantitative muscle force measurements swiftly is challenging. While fast observational tools offer quick assessments, they lack quantitative data over time, unlike laboratory-based assessments which provide detailed but time-consuming data. This work aims to achieve rapid and quantitative measurements through wearable sensors, musculoskeletal modeling, and signal-processing techniques. Three studies are presented. First an automated muscle localization algorithm combined with EMG-embedded garment and EMG-driven musculoskeletal modeling for the estimation of ankle torque during diverse dynamic tasks. Secondly we present an enhanced automated muscle localization algorithm that generalizes across anatomies and control strategies, involving both healthy and post-stroke individuals. Finally, we used Inertial motion unit (IMU) sensors to replace laboratory-based technologies while still enabling non-invasive estimation of musculoskeletal parameters. In conclusion, this dissertation shows that wearable and automated technologies offer viable alternatives to standard laboratory techniques, saving experimental time and facilitating the integration of advanced modeling pipelines in clinical and other settings.
Download Thesis